Data Scientists: Take Data Wrangling and Prep Out of Your Job Description
It is a well-known industry problem that data scientists typically spend at least 80% of their time finding and prepping data instead of analyzing it. The IBM study that originally published this statistic dates to even before most organizations adopted separate best-of-breed applications in functional business units. Typically, there is not one central data source used by the entire company, but instead there exist multiple data silos throughout an organization due to decentralized purchasing and adoption of applications best suited for a particular use or business function. This means that now data scientists must cobble together data from multiple sources, often having separate “owners,” wrangle IT and the various data owners to extract and get the data to their analytics, and then make it usable. Having Data Analysts and Data Scientists work on building data pipelines is not only a complex technical problem but also a complex political problem.
To visualize analytics results, a data analyst is not expected to build a new dashboard application. Instead, Tableau, Power BI and other out-of-the-box solutions are used. So why require a data analyst to build data pipelines instead of using an out-of-the-box solution that can build, integrate, and wrangle pipelines of data in a matter of minutes? Because it saves time, money, and angst to:
- Automate data pipeline building and wrangling for analytics-ready data to be delivered to the analysts;
- Automatically create a real-time stream of integrated, munched and cleansed data, and feed it into analytics in order to have current and trusted data available for immediate decision-making;
- Have automated data profiling to give analysts insights about metadata, outliers, and ranges and automatically detect data quality issues;
- Have built-in data governance and lineage so that a single piece of data can be tracked to its source; and
- Automatically detect when changes are made to source data and update the analytics without blowing up algorithms.
Building data pipelines is not the best use of a data scientist’s time. Data scientists instead need to spend their highly compensated time developing models, examining statistical results, comparing models, checking interpretations, and iterating on the models. This is particularly important given that there exists a labor shortage of these highly skilled workers in both North America and Europe and adding more FTEs into the cost of analytics projects makes them harder for management to justify.
According to David Cieslak, Ph.D., Chief Data Scientist at Aunalytics, without investment in automation and data democratization, the rate at which one can execute on data analytics use cases — and realize the business value — is directly proportionate to the number of data engineers, data scientists, and data analysts hired. Using a data platform with built-in data integration and cleansing to automatically create analytics-ready pipelines of business information allows data scientists to concentrate on creating analytical results. This enables companies to rapidly build insights based upon trusted data and deliver those insights to clients in a fraction of the time that it would take if they had to manually wrangle the data.
Our Solution: Aunsight Golden Record
Aunsight™ Golden Record turns siloed data from disparate systems into a single source of truth across your enterprise. Powered with data accuracy, our cloud-native platform cleanses data to reduce errors, and Golden Record as a Service matches and merges data together into a single source of accurate business information – giving you access to consistent trusted data across your organization in real-time. With this self-service offering, unify all your data to ensure enterprise-wide consistency and better decision making.
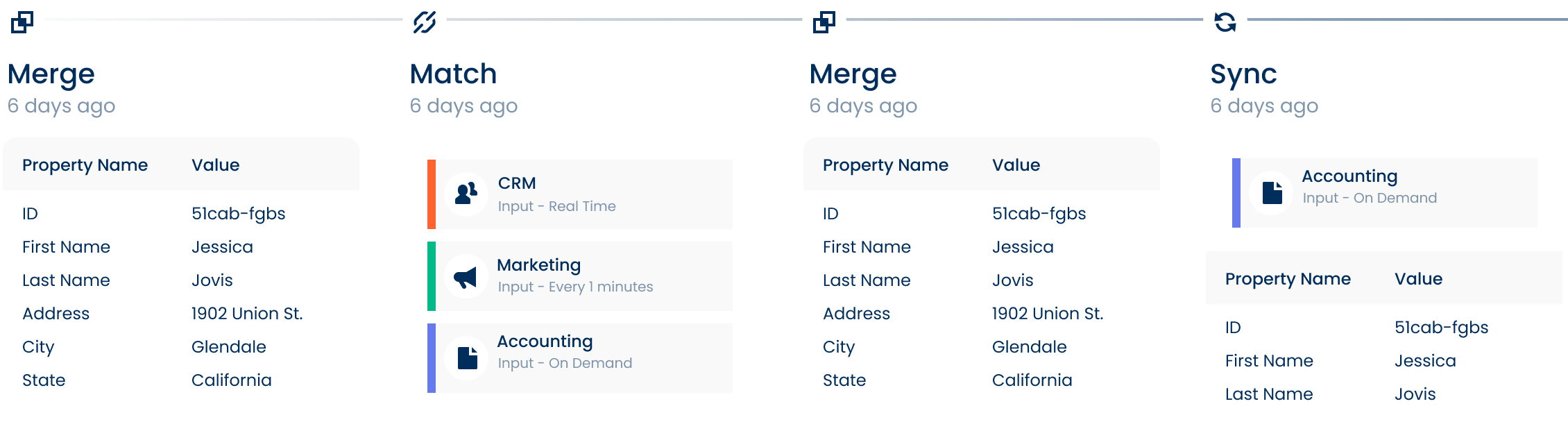